LiSA-LOC
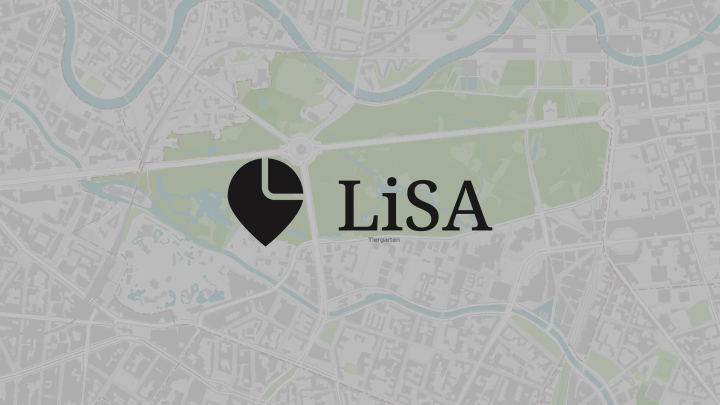
Objective
To develop a robust and accurate localization method for autonomous driving by leveraging the complementary strengths of satellite imagery and LiDAR data, thereby mitigating the limitations inherent in traditional point cloud map-based localization.
Solution
We propose a novel approach that extracts building edge information from both geo-referenced satellite imagery (enhanced by OpenStreetMap data and machine learning-based segmentation) and LiDAR point clouds. These extracted edges are represented as geo-referenced point clouds. An initial pose estimate is obtained from a GNSS/INS system. Subsequently, a sensing and filtering module isolates building edge points from the LiDAR data, and these points are registered against the edge-based point cloud map derived from satellite imagery using the Generalized Iterative Closest Point (GICP) algorithm to achieve accurate localization in a global frame.
Conclusion
The proposed method demonstrates promising localization performance across various evaluation datasets. The achieved average absolute pose error of 2.5 meters, along with average translational and rotational relative pose errors of 0.09 meters and 0.003 radians, respectively, indicates a high degree of consistency with the ground truth trajectory. The results suggest that the primary source of localization error stems from inaccuracies present in the generated point cloud map, highlighting the potential for further improvement by refining the mapping process or the integration of satellite-derived information directly into map construction.